AI Agent Reinventing E-commerce Customer Service: A Practical Analysis Based on the Coze Platform
The traditional e-commerce customer service system relies on the cooperation between intelligent customer service systems and human teams, but this model often faces efficiency bottlenecks and experience pain points. Although big model technology has been developed for several years, most AI customer service is still limited to assistance based on predefined processes (SOP mode) or simple human-machine collaboration (SOP mode).Copilot
(Mode).
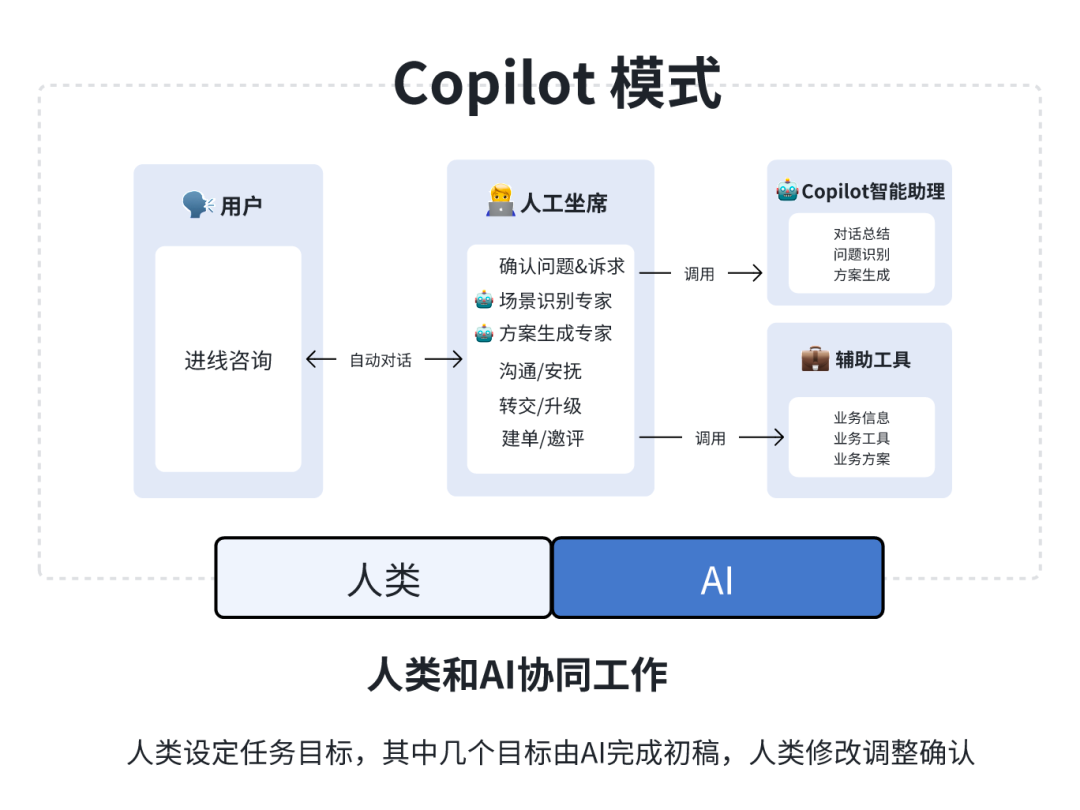
Problems such as "pre-configured to reply", "mechanical one-round Q&A", "generating inaccurate information to mislead users", and "inability to accurately recognize the user's intent, leading to irrelevant answers" significantly limit the effectiveness of AI customer service. The effectiveness of AI customer service is significantly limited by issues such as the need for pre-configuration to respond, "mechanical one-round Q&A," "misleading users by generating inaccurate information," and "inability to accurately recognize the user's intent, leading to unintelligible answers. These limitations are due to their lack of true autonomy and ability to understand and plan complex conversations. In order to break through these limitations, the industry has begun to explore the feasibility of AI customer service based on Agent
AI customer service solutions in mode. Taking the Jitterbug e-commerce customer service team as an example, it utilizes the Coze
Platform construction Agent
, exploring a new paradigm for human-computer collaboration.
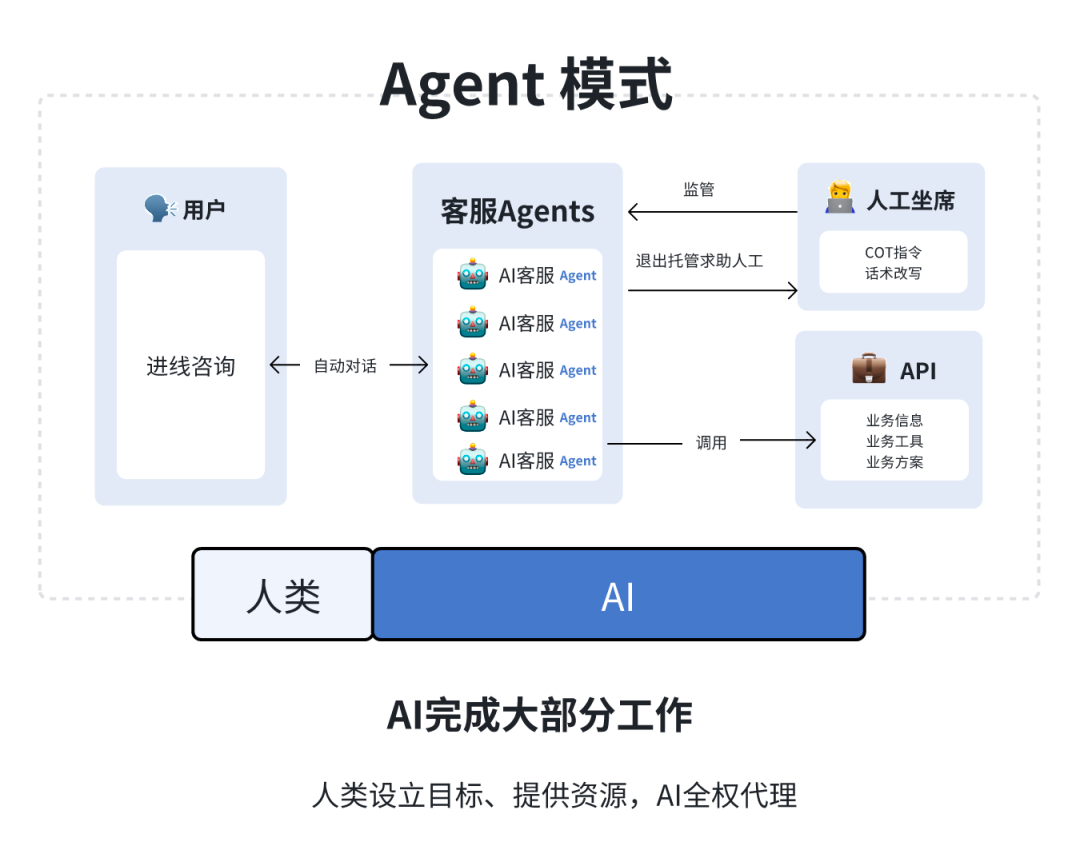
Improve efficiency:Coze
Two application models of Agent in customer service scenarios
The traditional e-commerce customer service process (user query -> robot response -> transfer to manual) is likely to cause users to wait for a long time and affect the experience when the volume of inquiries is high during the peak period; at the same time, manual handling of a large number of repetitive inquiries is not only costly, but also difficult to improve the efficiency. Based on the big model and Agent
The exploration of the technology has resulted in two main application models:

Agent
Direct customer service: by AIAgent
Provide services directly to customers. By providing services inCoze
Platform buildingAgent
intelligences, and trained with common problems and solutions accumulated in historical dialog data.Agent
Ability to respond quickly to customer inquiries. More importantly.Agent
With the ability to continuously learn, its answer accuracy improves with the number of times it is used. In dealing with certain standard or repetitive question scenarios, this model has the potential to completely replace manual customer service and significantly reduce operational costs.Agent
Response + Manual Supervision: This is a more general model of transition or collaboration. It consists mainly ofAgent
The model generates response suggestions, which are then reviewed and confirmed by a live customer service agent. Only when the model response is risky or unable to deal with complex and emotional questions will manual intervention be made. In order to quantitatively assess the performance of the model and the necessity of manual intervention, indicators such as "effective intervention rate" are introduced to judge the quality of AI replies and the reasonableness of manual correction. This model ensures service quality and provides valuable feedback data for the continuous optimization of the AI model.
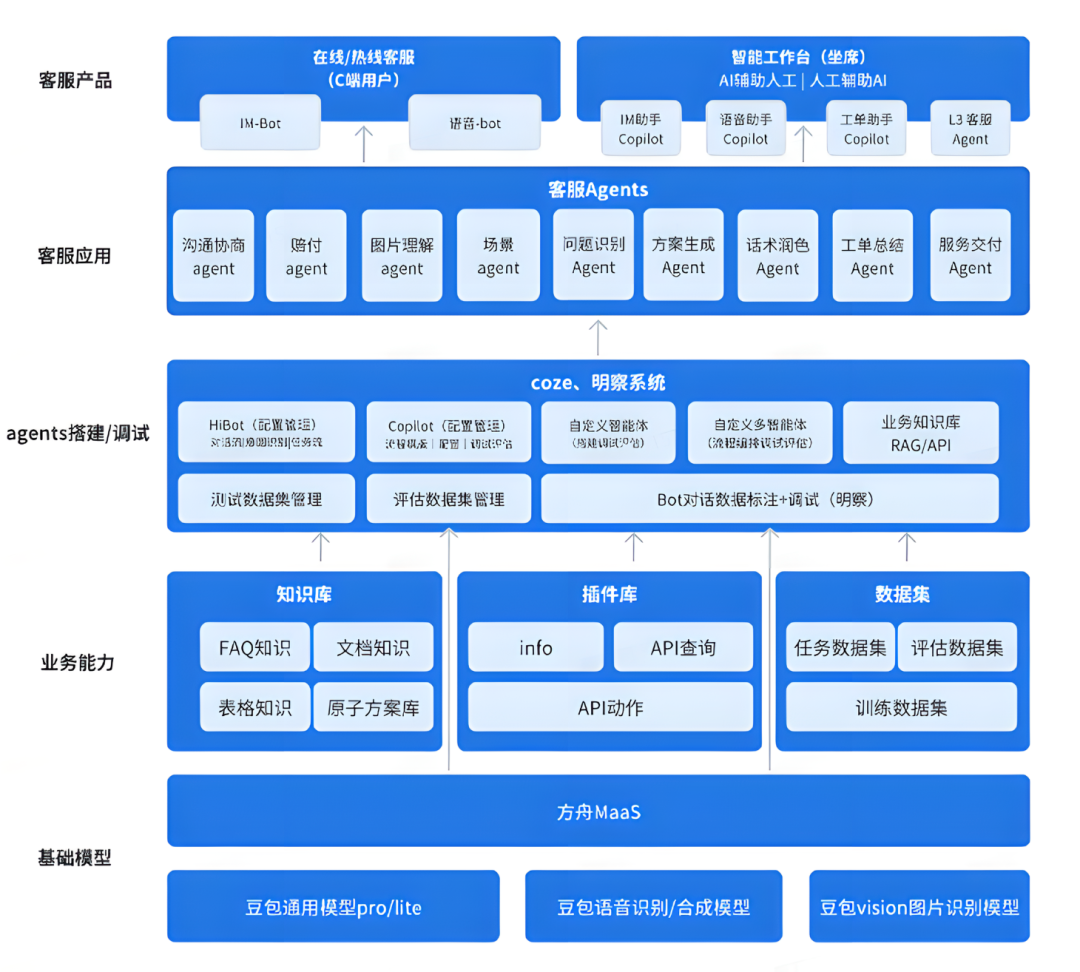
Implementation Path: Deconstructing and Constructing Customer Service Agent
The Jitterbug e-commerce team is based on Coze
platform practice, especially in after-sales customer service scenarios, which builds the Agent
has been used as a template in the Coze
The platform store is open for other enterprises or developers to use for free. The template is designed to deal with common problems such as logistics, payment, after-sales, etc., providing a referable idea for building intelligent customer service to replace manual labor. Users can according to their own business needs, the Agent
and related workflows for customized modifications.
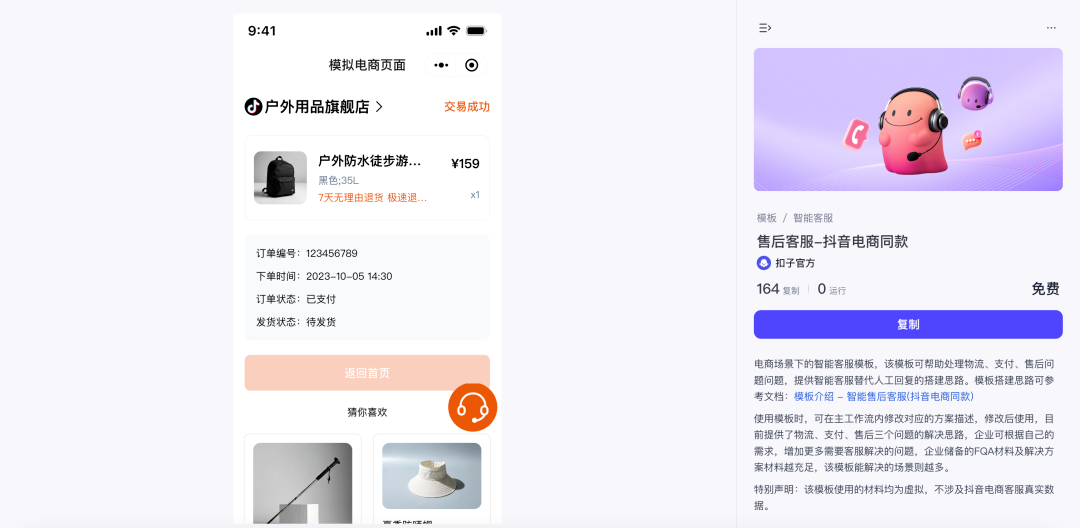
Get this Agent
The address of the template is:https://www.coze.cn/s/uM1e-yIzEzo/
The core idea of the program is to abstract the complex traditional customer service functions into intelligent service units that can be data-driven and dispatched, and to build an intelligent body system that can autonomously execute service processes. Its specific implementation path mainly includes two key steps:
Step 1: Deconstruct the customer service process
Break down the entire customer service interaction process into a series of well-defined and executable subtasks or modules.

Step 2: Workflow Architecture Design
Based on the deconstructed process, the design Agent
of the workflow architecture, which typically contains the following types of nodes:
- Main Workflow: Responsible for stringing together the entire service link, performing initial analysis of the session (e.g., determining user intent, session stage), and routing tasks to the appropriate
Agent
Nodes. Agent
Agent Node: EachAgent
Nodes take on a separate customer service function, such as opening greetings and closing remarks, problem clarification, intent recognition and scenario routing, solution determination, negotiation and execution of solutions. This modular design allowsAgent
Easier to manage and expand.- Configuration Node: For management and configuration
Agent
External information and parameters required for operation, such as knowledge base access, conditional configuration of judgment logic, parameters of the Large Language Model (LLM) (e.g., temperature, maximum Token (number) settings, etc. - Code Node: Used to perform specific functions that need to be programmed, such as interaction with internal systems (e.g., IM communication interfaces, ordering systems), user profile parsing, A/B test parameter acquisition, etc. This provides
Agent
Provides the ability to interface with complex external environments.
Coze
Platform Enablement: Low-Code Meets Intelligent
Coze
Platform for building a strong customer service Agent
Key support is provided and its strengths are reflected in:
- Self-learning capability with near-zero configuration::
Agent
Out-of-the-box, no tedious pre-configuration is required, and it has the ability to learn and optimize on its own from interactions. - Precise Intelligent Response: It can combine multi-dimensional data such as product attributes and historical user conversations to more accurately recognize user intent and generate relevant responses.
- Deep decision making and scheduling: Supporting the handling of more complex customer scenarios through multi-dimensional analysis and reasoning, and enabling multiple
Agent
intelligent collaboration and task scheduling between them. This goes beyond traditional customer service bots based on rules or simple intent recognition. - Emotional Intelligence Sensing: It is able to identify the user's emotional changes in the conversation in real time and adjust the reply strategy accordingly, for example, to appease the user in time when he/she expresses dissatisfaction and optimize the service experience.
- Intelligent dialog guidance: Supports more natural multi-round interactions, can actively track changes in user needs, and guide conversations toward problem solving, providing deeper solutions rather than simple Q&A.
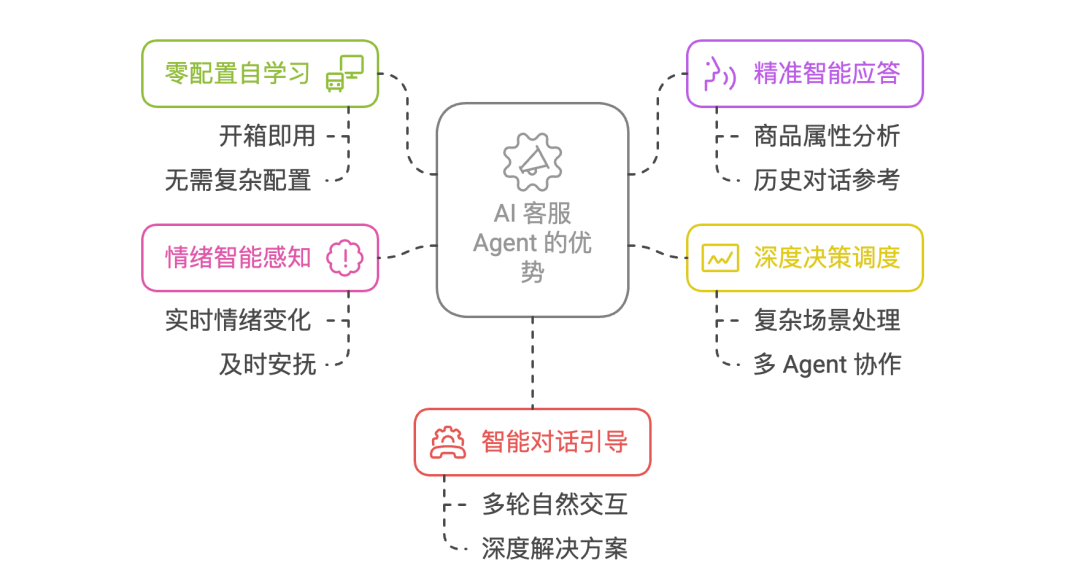
Application Practice: More Enterprise Exploration
In addition to the Shake Shack e-commerce team, other businesses are utilizing the Coze
The platform builds customized Agent
customer service system. For example, Explore Domain Technology is based on Coze
The customized e-commerce intelligences are able to quickly locate product characteristics, promotional campaign information, and historical conversation records from the knowledge base to generate personalized marketing discourse to enhance product appeal and conversion rates. In a multi-round dialog, the Agent
It can also be combined with information about the store's postage policy, shipping insurance rules, and coupon strategies to answer consumer concerns, build trust, and assist with purchase decisions.
on the basis of Coze
Example of built e-commerce intelligence
Customer Application Showcase: Customer Service Agent
Performs the whole process from welcoming into the store, receiving inquiries, recommending sizes according to the user's body shape, guiding the wearing effect to assisting in completing the transaction.
liberalization Agent
Templates are a useful attempt to lower the technical threshold and accelerate industry adoption. However, theAgent
There are still challenges to the widespread adoption of the model, including the reliance on high-quality data, the ability to process complex or non-standard scenarios, and service boundaries and ethical considerations in fully automated scenarios.
Despite the evolution of technology, the core goal of the service - to effectively solve user problems - remains the same.Coze
platforms and their Agent
The ability to build new possibilities for e-commerce and other areas to explore smarter and more efficient service models.
© Copyright notes
The article is copyrighted and should not be reproduced without permission.
Related posts
No comments...