Mastering Crawl4AI: Preparing High-Quality Web Data for LLM and RAG
Traditional web crawler frameworks are versatile, but often require additional cleansing and formatting when processing data, which makes their integration with Large Language Models (LLMs) relatively complex. The output of many tools (e.g., raw HTML
or unstructured JSON
) contains a lot of noise and is not suitable for direct use in scenarios such as Retrieval Augmented Generation (RAG), as it would degrade LLM
Efficiency and accuracy of processing.
Crawl4AI offers a different kind of solution. It focuses on directly generating clean, structured Markdown
Formatted content. This format preserves the semantic structure of the original text (e.g., headings, lists, code blocks) while intelligently removing extraneous elements such as navigation, advertisements, footers, etc., making it ideal for use as a LLM
inputs or for building high-quality RAG
Dataset.Crawl4AI
is a completely open source project that uses no API
The key is also not set at a pay-per-view threshold.
Installation and configuration
Recommended Use uv Create and activate a separate Python
virtual environment to manage project dependencies.uv
is a program based on the Rust
Developed Emerging Python
package manager, with its significant speed advantage (often over the pip
(3-5 times faster) and efficient parallel dependency resolution.
# 创建虚拟环境
uv venv crawl4ai-env
# 激活环境
# Windows
# crawl4ai-env\Scripts\activate
# macOS/Linux
source crawl4ai-env/bin/activate
After the environment is activated, use the uv
mounting Crawl4AI
Core library:
uv pip install crawl4ai
Once the installation is complete, run the initialization command, which will take care of installing or updating the Playwright
Required browser drivers (e.g. Chromium
) and perform environmental inspections.Playwright
It's one of those things that's made up of Microsoft
developed browser automation libraries.Crawl4AI
Use it to simulate real user interactions so that you can handle dynamically loading content in the JavaScript
Heavy website.
crawl4ai-setup
If you encounter problems related to the browser driver, you can try to install it manually:
# 手动安装 Playwright 浏览器及依赖
python -m playwright install --with-deps chromium
As needed, this can be done by uv
Installation of expansion packs containing additional features:
# 安装文本聚类功能 (依赖 PyTorch)
uv pip install "crawl4ai[torch]"
# 安装 Transformers 支持 (用于本地 AI 模型)
uv pip install "crawl4ai[transformer]"
# 安装所有可选功能
uv pip install "crawl4ai[all]"
Basic Crawling Example
that amount or less Python
The script demonstrates the Crawl4AI
The basic usage of the Markdown
The
import asyncio
from crawl4ai import AsyncWebCrawler
async def main():
# 初始化异步爬虫
async with AsyncWebCrawler() as crawler:
# 执行爬取任务
result = await crawler.arun(
url="https://www.sitepoint.com/react-router-complete-guide/"
)
# 检查爬取是否成功
if result.success:
# 输出结果信息
print(f"标题: {result.title}")
print(f"提取的 Markdown ({len(result.markdown)} 字符):")
# 仅显示前 300 个字符作为预览
print(result.markdown[:300] + "...")
# 将完整的 Markdown 内容保存到文件
with open("example_content.md", "w", encoding="utf-8") as f:
f.write(result.markdown)
print(f"内容已保存到 example_content.md")
else:
# 输出错误信息
print(f"爬取失败: {result.url}")
print(f"状态码: {result.status_code}")
print(f"错误信息: {result.error_message}")
if __name__ == "__main__":
asyncio.run(main())
After executing this script, theCrawl4AI
will activate Playwright
The controlled browser accesses the specified URL
Execution page JavaScript
, then intelligently recognizes and extracts the main content areas, filters distracting elements, and ultimately generates clean Markdown
Documentation.
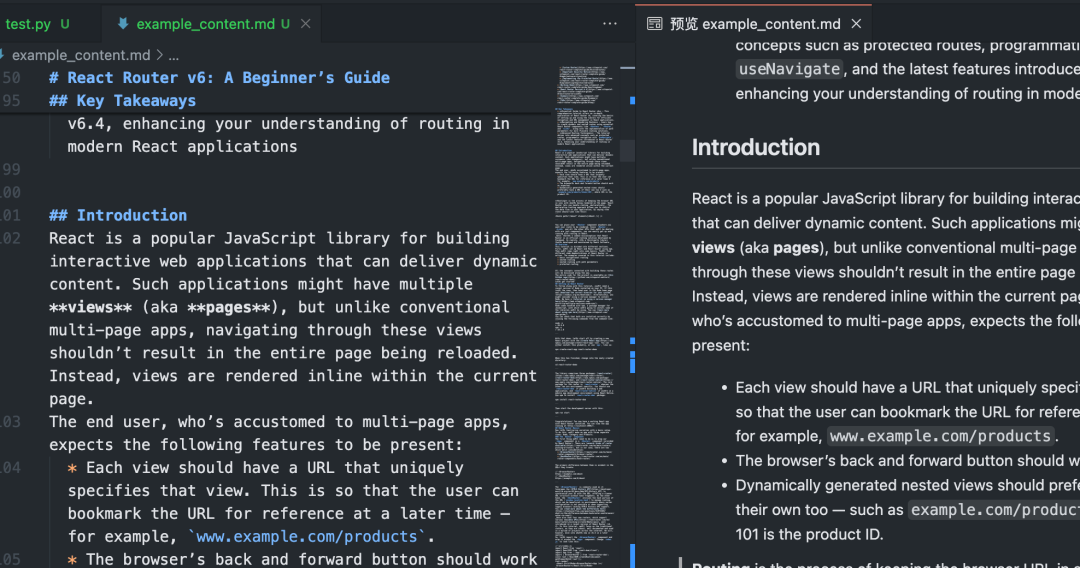
Batch and Parallel Crawling
process multiple URL
whenCrawl4AI
of parallel processing can dramatically increase efficiency. By configuring the CrawlerRunConfig
hit the nail on the head concurrency
parameter, which controls the number of pages processed simultaneously.
import asyncio
from crawl4ai import AsyncWebCrawler, BrowserConfig, CrawlerRunConfig, CacheMode
async def main():
urls = [
"https://example.com/page1",
"https://example.com/page2",
"https://example.com/page3",
# 添加更多 URL...
]
# 浏览器配置:无头模式,增加超时
browser_config = BrowserConfig(
headless=True,
timeout=45000, # 45秒超时
)
# 爬取运行配置:设置并发数,禁用缓存以获取最新内容
run_config = CrawlerRunConfig(
concurrency=5, # 同时处理 5 个页面
cache_mode=CacheMode.BYPASS # 禁用缓存
)
results = []
async with AsyncWebCrawler(browser_config=browser_config) as crawler:
# 使用 arun_many 进行批量并行爬取
# 注意:arun_many 需要将 run_config 列表传递给 configs 参数
# 如果所有 URL 使用相同配置,可以创建一个配置列表
configs = [run_config.clone(url=url) for url in urls] # 为每个URL克隆配置并设置URL
# arun_many 返回一个异步生成器
async for result in crawler.arun_many(configs=configs):
if result.success:
results.append(result)
print(f"已完成: {result.url}, 获取了 {len(result.markdown)} 字符")
else:
print(f"失败: {result.url}, 错误: {result.error_message}")
# 将所有成功的结果合并到一个文件
with open("combined_results.md", "w", encoding="utf-8") as f:
for i, result in enumerate(results):
f.write(f"## {result.title}\n\n")
f.write(result.markdown)
f.write("\n\n---\n\n")
print(f"所有成功内容已合并保存到 combined_results.md")
if __name__ == "__main__":
asyncio.run(main())
take note of: The above code uses the arun_many
method, which is the recommended way to handle large lists of URLs, rather than looping through calls to the arun
More efficient.arun_many
A list of configurations is required, each corresponding to a URL
. If all URL
Using the same basic configuration, the clone()
method creates a copy and sets a specific URL
The
Structured Data Extraction (Selector Based)
apart from Markdown
(math.) genusCrawl4AI
Can also use CSS
Selector or XPath
Extracts structured data, ideal for sites with regular data formats.
import asyncio
import json
from crawl4ai import AsyncWebCrawler, ExtractorConfig
async def main():
# 定义提取规则 (CSS 选择器)
extractor_config = ExtractorConfig(
strategy="css", # 明确指定策略为 CSS
rules={
"products": {
"selector": "div.product-card", # 主选择器
"type": "list",
"properties": {
"name": {"selector": "h2.product-title", "type": "text"},
"price": {"selector": ".price span", "type": "text"},
"link": {"selector": "a.product-link", "type": "attribute", "attribute": "href"}
}
}
}
)
async with AsyncWebCrawler() as crawler:
result = await crawler.arun(
url="https://example-shop.com/products",
extractor_config=extractor_config
)
if result.success and result.extracted_data:
extracted_data = result.extracted_data
with open("products.json", "w", encoding="utf-8") as f:
json.dump(extracted_data, f, ensure_ascii=False, indent=2)
print(f"已提取 {len(extracted_data.get('products', []))} 个产品信息")
print("数据已保存到 products.json")
elif not result.success:
print(f"爬取失败: {result.error_message}")
else:
print("未提取到数据或提取规则匹配失败")
if __name__ == "__main__":
asyncio.run(main())
This approach does not require LLM
Intervention, which is low cost and fast, is suitable for scenarios where the target element is clear.
AI-enhanced data extraction
For pages with a complex structure or no fixed pattern, you can utilize the LLM
Perform intelligent extraction.
import asyncio
import json
from crawl4ai import AsyncWebCrawler, BrowserConfig, AIExtractorConfig
async def main():
# 配置 AI 提取器
ai_config = AIExtractorConfig(
provider="openai", # 或 "local", "anthropic" 等
model="gpt-4o-mini", # 使用 OpenAI 的模型
# api_key="YOUR_OPENAI_API_KEY", # 如果环境变量未设置,在此提供
schema={
"type": "object",
"properties": {
"article_summary": {"type": "string", "description": "A brief summary of the article."},
"key_topics": {"type": "array", "items": {"type": "string"}, "description": "List of main topics discussed."},
"sentiment": {"type": "string", "enum": ["positive", "negative", "neutral"], "description": "Overall sentiment of the article."}
},
"required": ["article_summary", "key_topics"]
},
instruction="Extract the summary, key topics, and sentiment from the provided article text."
)
browser_config = BrowserConfig(timeout=60000) # AI 处理可能需要更长时间
async with AsyncWebCrawler(browser_config=browser_config) as crawler:
result = await crawler.arun(
url="https://example-news.com/article/complex-analysis",
ai_extractor_config=ai_config
)
if result.success and result.ai_extracted:
ai_extracted = result.ai_extracted
print("AI 提取的数据:")
print(json.dumps(ai_extracted, indent=2, ensure_ascii=False))
# 也可以选择保存到文件
# with open("ai_extracted_data.json", "w", encoding="utf-8") as f:
# json.dump(ai_extracted, f, ensure_ascii=False, indent=2)
elif not result.success:
print(f"爬取失败: {result.error_message}")
else:
print("AI 未能提取所需数据。")
if __name__ == "__main__":
asyncio.run(main())
AI extraction offers great flexibility to understand content and generate structured output on demand, but incurs additional API
Cost of invocation (if using cloud services) LLM
) and processing time. Select the local model (e.g. Mistral
, Llama
) can reduce costs and protect privacy, but have local hardware requirements.
Advanced Configurations and Tips
Crawl4AI
Provides a wealth of configuration options to deal with complex scenarios.
Browser Configuration (BrowserConfig
)
BrowserConfig
Controls the startup and behavior of the browser itself.
from crawl4ai import BrowserConfig
config = BrowserConfig(
browser_type="firefox", # 使用 Firefox 浏览器
headless=False, # 显示浏览器界面,方便调试
user_agent="MyCustomCrawler/1.0", # 设置自定义 User-Agent
proxy_config={ # 配置代理服务器
"server": "http://proxy.example.com:8080",
"username": "proxy_user",
"password": "proxy_password"
},
ignore_https_errors=True, # 忽略 HTTPS 证书错误 (开发环境常用)
use_persistent_context=True, # 启用持久化上下文
user_data_dir="./my_browser_profile", # 指定用户数据目录,用于保存 cookies, local storage 等
timeout=60000, # 全局浏览器操作超时 (毫秒)
verbose=True # 打印更详细的日志
)
# 在初始化 AsyncWebCrawler 时传入
# async with AsyncWebCrawler(browser_config=config) as crawler:
# ...
Crawl the runtime configuration (CrawlerRunConfig
)
CrawlerRunConfig
Control Single arun()
maybe arun_many()
The specific behavior of the call.
from crawl4ai import CrawlerRunConfig, CacheMode
run_config = CrawlerRunConfig(
cache_mode=CacheMode.READ_ONLY, # 只读缓存,不写入新缓存
check_robots_txt=True, # 检查并遵守 robots.txt 规则
wait_until="networkidle", # 等待网络空闲再提取,适合JS动态加载内容
wait_for="css:div#final-content", # 等待特定 CSS 选择器元素出现
js_code="window.scrollTo(0, document.body.scrollHeight);", # 页面加载后执行 JS 代码 (例如滚动到底部触发加载)
scan_full_page=True, # 尝试自动滚动页面以加载所有内容 (用于无限滚动)
screenshot=True, # 截取页面截图 (结果在 result.screenshot,Base64编码)
pdf=True, # 生成页面 PDF (结果在 result.pdf,Base64编码)
word_count_threshold=50, # 过滤掉少于 50 个单词的文本块
excluded_tags=["header", "nav", "footer", "aside"], # 从 Markdown 中排除特定 HTML 标签
exclude_external_links=True # 不提取外部链接
)
# 在调用 arun() 或创建配置列表给 arun_many() 时传入
# result = await crawler.arun(url="...", config=run_config)
Handling JavaScript and Dynamic Content
thanks to Playwright
(math.) genusCrawl4AI
Handles dependencies well JavaScript
Rendered website. Key Configuration:
wait_until
: Set to"networkidle"
maybe"load"
It's usually a bit more efficient than the default"domcontentloaded"
More suitable for dynamic pages.wait_for
: wait for a specific element orJavaScript
Conditions met.js_code
: Execute customizations after the page loadsJavaScript
, such as clicking buttons and scrolling pages.scan_full_page
: Automatically handle common infinite scrolling pages.delay_before_return_html
: Add a short delay before extraction to ensure that all scripts are executed.
Error handling and debugging
- probe
result.success
: Be sure to check this property after each crawl. - ferret out
result.status_code
cap (a poem)result.error_message
:: Get the reason for the failure. - set up
headless=False
: InBrowserConfig
You can observe the browser operation and diagnose the problem visually. - start using
verbose=True
: InBrowserConfig
in the settings to get a more detailed runtime log. - utilization
try...except
: Packagesarun()
maybearun_many()
call that captures a possiblePython
Exception.
import asyncio
from crawl4ai import AsyncWebCrawler, BrowserConfig
async def debug_crawl():
# 启用调试模式:显示浏览器,打印详细日志
debug_browser_config = BrowserConfig(headless=False, verbose=True)
async with AsyncWebCrawler(browser_config=debug_browser_config) as crawler:
try:
result = await crawler.arun(url="https://problematic-site.com")
if not result.success:
print(f"Crawl failed: {result.error_message} (Status: {result.status_code})")
else:
print("Crawl successful.")
# ... process result ...
except Exception as e:
print(f"An unexpected error occurred: {e}")
if __name__ == "__main__":
asyncio.run(debug_crawl())
observance robots.txt
When performing a web crawl, respect the site's robots.txt
Documentation is basic netiquette and prevents IP blocking.Crawl4AI
It can be handled automatically.
exist CrawlerRunConfig
set up in check_robots_txt=True
::
respectful_config = CrawlerRunConfig(
check_robots_txt=True
)
# result = await crawler.arun(url="https://example.com", config=respectful_config)
# if not result.success and result.status_code == 403:
# print("Access denied by robots.txt")
Crawl4AI
Automatically downloaded, cached and parsed robots.txt
file, if the rule prohibits access to the target URL
(math.) genusarun()
will fail.result.success
because of False
(math.) genusstatus_code
This is usually 403 with the appropriate error message.
Session Management (Session Management
)
For multi-step operations that require logging in or maintaining state (e.g., form submission, paged navigation), session management can be used. This can be accomplished by adding a new session manager to the CrawlerRunConfig
Specify the same session_id
The following is an example of a program that can be used in more than one arun()
The same browser page instance is reused between calls, preserving the cookies
cap (a poem) JavaScript
Status.
import asyncio
from crawl4ai import AsyncWebCrawler, CrawlerRunConfig, CacheMode
async def session_example():
async with AsyncWebCrawler() as crawler:
session_id = "my_unique_session"
# Step 1: Load login page (hypothetical)
login_config = CrawlerRunConfig(session_id=session_id, cache_mode=CacheMode.BYPASS)
await crawler.arun(url="https://example.com/login", config=login_config)
print("Login page loaded.")
# Step 2: Execute JS to fill and submit login form (hypothetical)
login_js = """
document.getElementById('username').value = 'user';
document.getElementById('password').value = 'pass';
document.getElementById('loginButton').click();
"""
submit_config = CrawlerRunConfig(
session_id=session_id,
js_code=login_js,
js_only=True, # 只执行 JS,不重新加载页面
wait_until="networkidle" # 等待登录后跳转完成
)
await crawler.arun(config=submit_config) # 无需 URL,在当前页面执行 JS
print("Login submitted.")
# Step 3: Crawl a protected page within the same session
protected_config = CrawlerRunConfig(session_id=session_id, cache_mode=CacheMode.BYPASS)
result = await crawler.arun(url="https://example.com/dashboard", config=protected_config)
if result.success:
print("Successfully crawled protected page:")
print(result.markdown[:200] + "...")
else:
print(f"Failed to crawl protected page: {result.error_message}")
# 清理会话 (可选,但推荐)
# await crawler.crawler_strategy.kill_session(session_id)
if __name__ == "__main__":
asyncio.run(session_example())
More advanced session management includes exporting and importing the browser's storage state (cookies
, localStorage
), allowing login to be maintained between script runs.
Crawl4AI
Provides a powerful and flexible feature set that, with proper configuration, can efficiently and reliably extract the required information from a variety of websites and prepare high-quality data for downstream AI applications.
© Copyright notes
The article is copyrighted and should not be reproduced without permission.
Related posts
No comments...