Step Star releases Step R-mini! Reasoning modeling will never be literature-biased again!
this is jumping stars The first inference model of the Step family of models.
OpenAI o1-like inference models are finally rolling up in the country.
Just now, Step Star, a member of "Six Little Tigers", released the latest generation model -- Step Reasoner mini ("Step R-mini" for short). This is the first reasoning model of Step series model family.
The new model excels at proactive planning, experimentation, and reflection, and is able to provide accurate and reliable responses to users through slow-thinking and iteratively validated logic mechanisms.
Moreover, it excels at solving complex problems such as logical reasoning, code and math through its extra-long reasoning ability, as well as general-purpose fields such as literary creation. Or 'both literature and science' according to the Order Leaping Star's own words.
According to benchmark data published by Step Star, the Step R-mini achieves SOTA on both math benchmarks, AIME 2024 and Math500, with Math500 scoring 2 points more than the o1-mini. the Step Reasoner mini also writes code well: it surpasses the o1-mini on the LiveCodeBench LiveCodeBench. It outperforms o1-preview on code tasks.
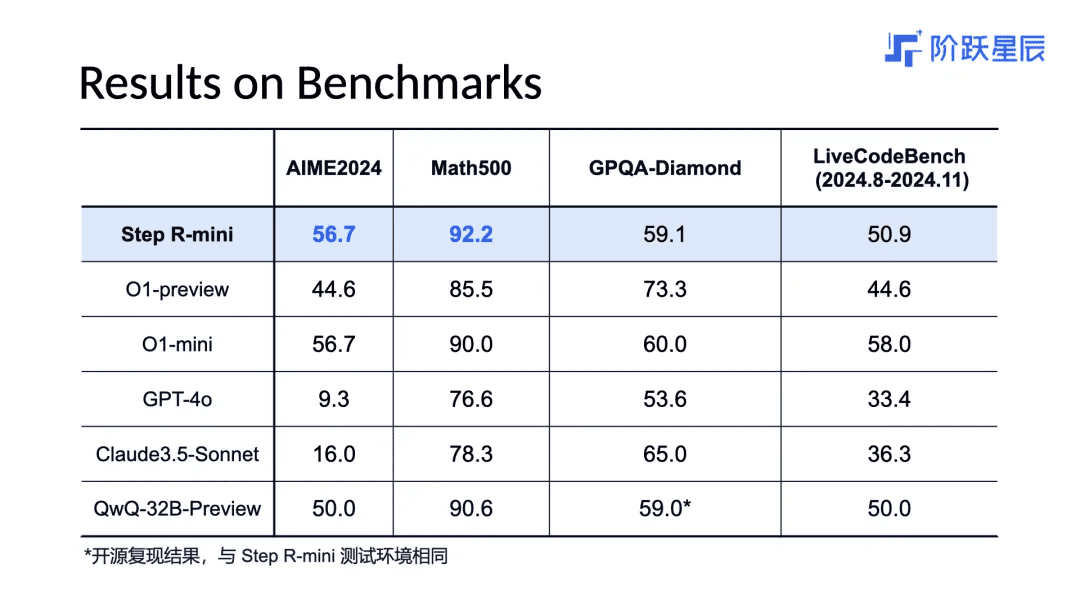
How does it all work? The heart of the machine learned from the people involved in Step Star that the new model has a high "RL" content, so the generalization is better. In addition, they also scaled in many aspects such as data quality, computation at test time, and model size, and once again verified that the Scaling Law The validity of the
In addition to the verbal reasoning model, they are also building a visual reasoning model that is capable of multimodal reasoning. Moreover, the source emphasized that this multimodal reasoning model "is really reasoning in the visual domain," i.e., reasoning on diagrams, "not just looking at diagrams but only reasoning in the textual domain."
It seems that Order Leaping Star has taken another step forward on its roadmap.
How is Step Reasoner mini made?
According to Step R-mini, the design and development of Step R-mini follows the current mainstream paradigm of reasoning model development. Specifically, it realizes 'slow thinking' by increasing the amount of computation in the reasoning phase and incorporating techniques such as thought chaining. The system is capable of proactive planning, experimentation and reflection based on the complexity of the task, thus providing accurate and reliable feedback through an iteratively validated logic mechanism.
According to Step Reasoner, one of the biggest highlights of Step Reasoner mini is that it realizes 'both literature and science'. Specifically, in addition to accurately answering math, code, and logical reasoning questions, Step Reasoner mini is also able to creatively perform literary content creation and daily chatting tasks. All of this is achieved through large-scale reinforcement learning training and the use of On-Policy reinforcement learning algorithms.
In addition, the improvement of model reasoning ability cannot be separated from Step Star's adherence to the principles of Scaling Law. This includes the following points:
- Scaling Reinforcement Learning: From imitation learning to reinforcement learning, from human preference to environmental feedback, StepStar adheres to Scaling Reinforcement Training, with reinforcement learning as the core training phase of model iteration.
- Scaling Data Quality: Data quality is the top priority. Under the premise of ensuring data quality, StepStar continues to expand the distribution and scale of data to provide a solid guarantee for reinforcement learning training.
- Scaling Test-Time Compute: Firmly implementing Training-Time Scaling along with Test-Time Scaling, Step Star found that the System 2 paradigm enables Step Reasoner mini to reach 50,000 think tokens on very complex task reasoning, thus enabling deep thinking. think tokens on very complex tasks, thus realizing deep thinking.
- Scaling model size: This is the most classic way of scaling. According to Step Star, insisting on model size Scaling is still the core of System-2, and we are already developing a smarter, more generalized Step Reasoner inference model with stronger synthesis ability.
How does a first-hand real-world test work?
Since Step Reasoner mini claims to be "literate", let's start by testing it with a literate question: In "Dreaming of Traveling to Tianmu Yinliu Farewell", which line is a number and its multiple in the same sentence? It's not a difficult question, but it requires the AI to remember and understand the poem as well as basic arithmetic. Although many people think the answer should be "The rooftop is 48,000 feet high, and this is the place that wants to fall to the southeast", in fact, 48,000 feet is the number of feet in the same line. But 48,000 is a separate number, and there are no multiples of it in this sentence, so it doesn't count. Step Reasoner mini also confirmed this in its reasoning, and finally found the correct answer: "The path of a thousand rocks and ten thousand turns is uncertain".
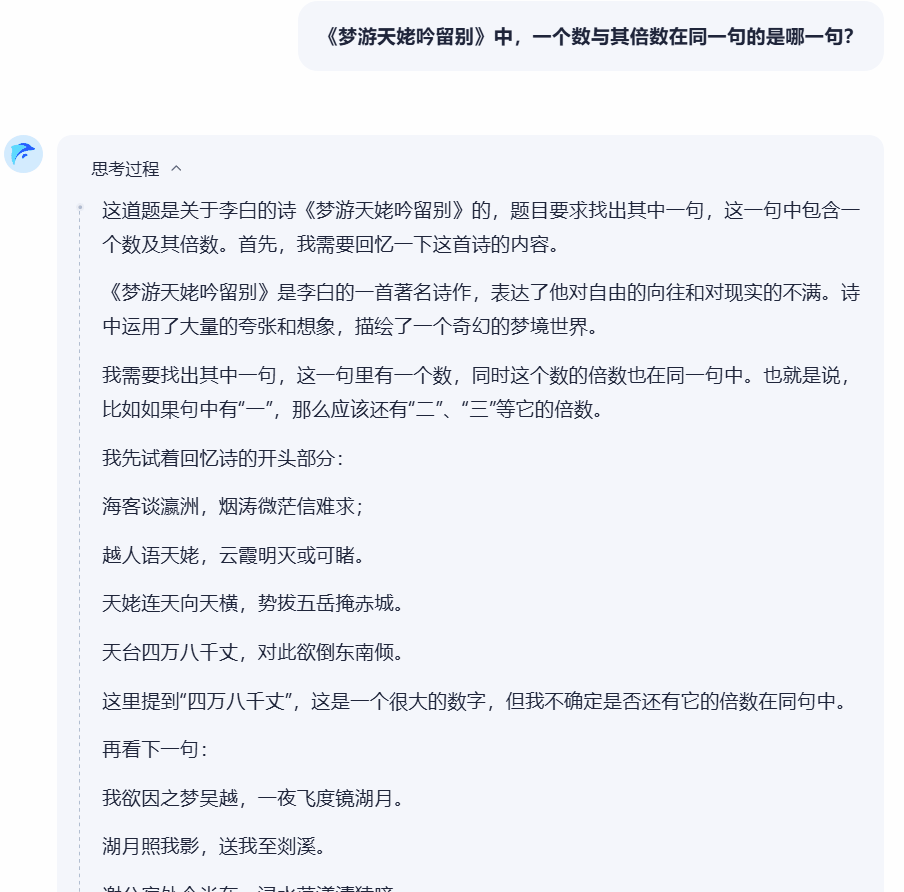
Here's a look at a 2024 high school math question:

As you can see, Step Reasoner mini performs a round of thinking to get the correct answer. It can also be seen that the model performs two more rounds of thinking after the first round of thinking to verify the correctness of the answer. In real-world testing, we found that if the answers from the first and second rounds did not match, Step Reasoner mini would continue to perform multiple rounds of thinking until it got the correct answer.
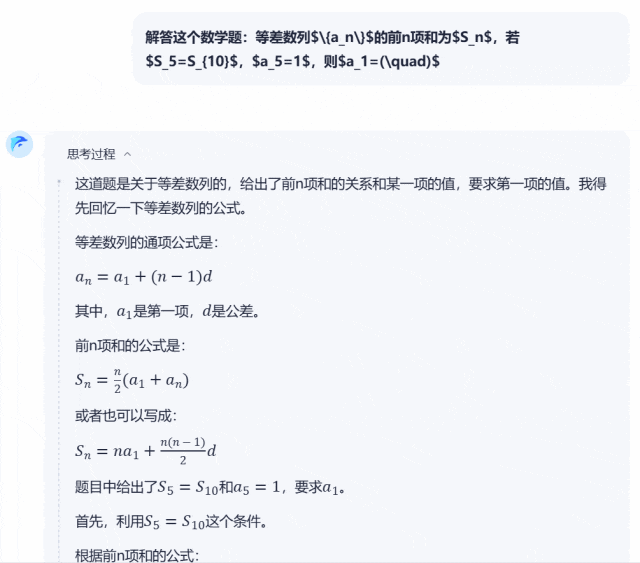
Next up is a logical reasoning question:
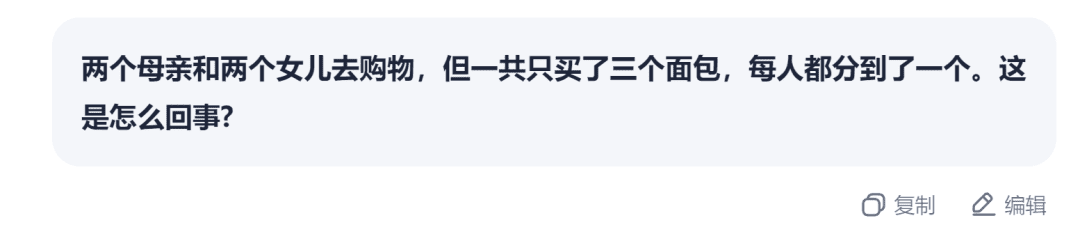
As you can see, Step Reasoner mini systematically organizes the relationships of several people in the question and presents the final answer in a very clear form.
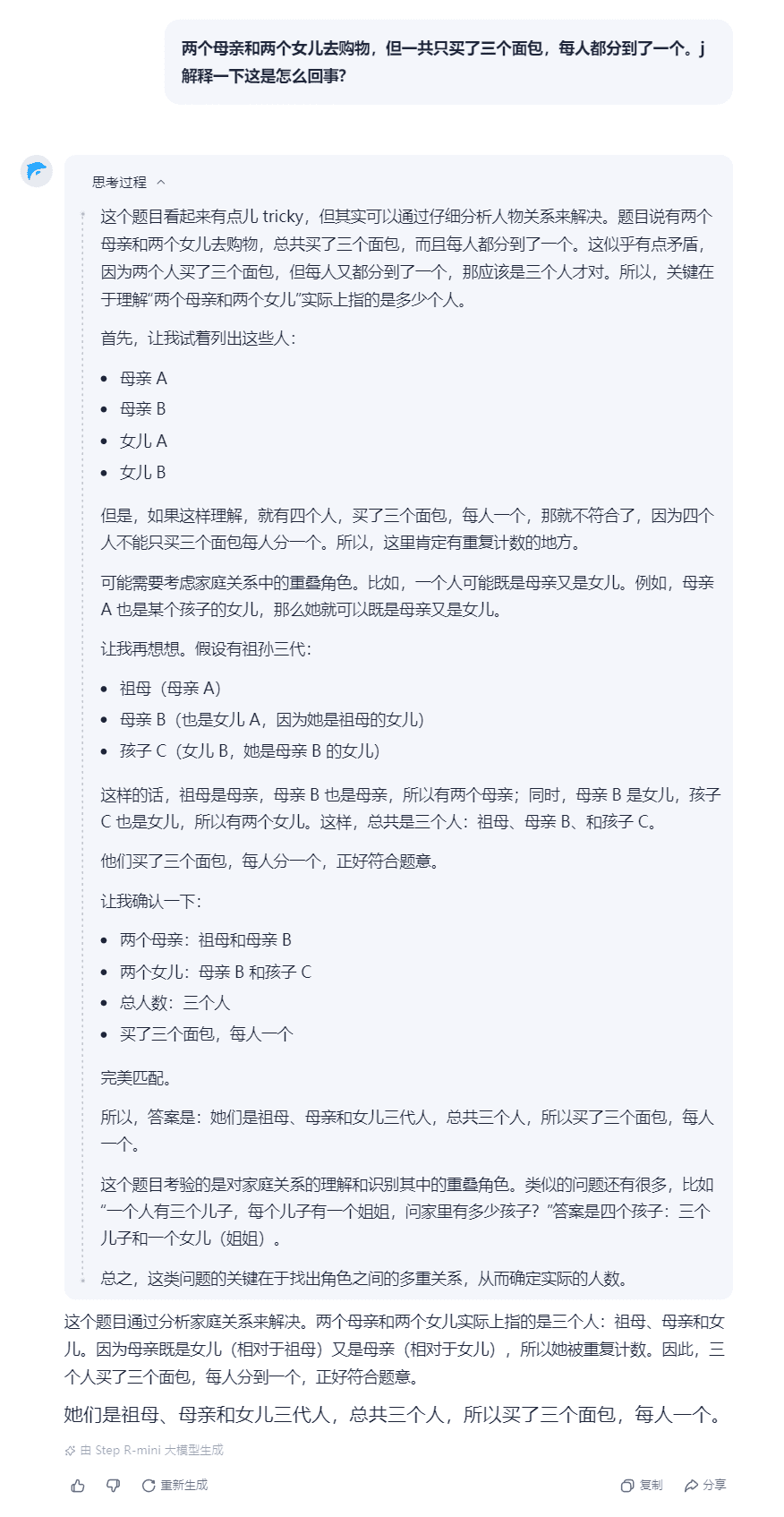
What about simple 'liberal arts' tasks? For example, if the model is asked to translate "I'm not in danger, I'm the danger." in a domineering way, what would it do? It turns out that Step Reasoner mini, after many attempts, finally found a rather overbearing translation: "I'm not in danger, I'm the danger." What's even more interesting is that in the process of thinking about it, it also referenced a similar line from The Dark Knight.
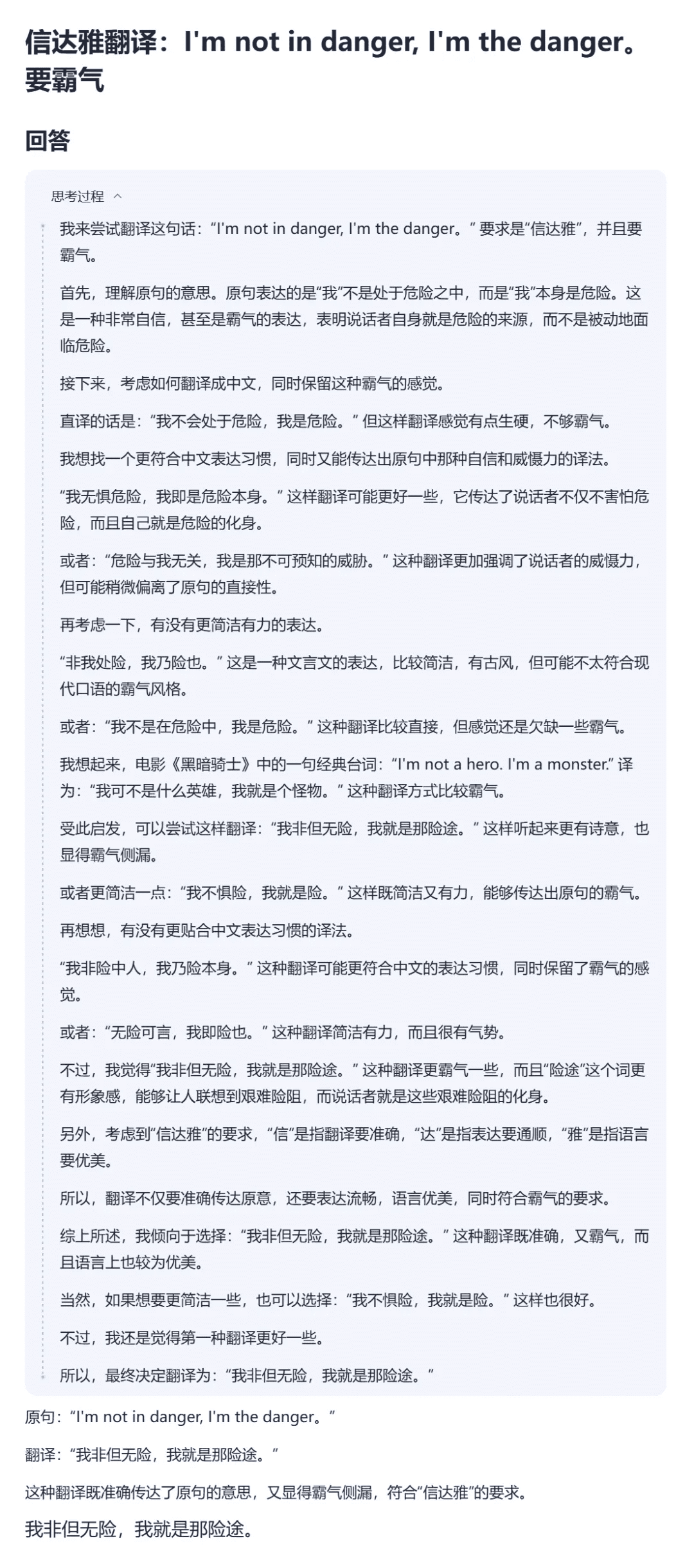
Also, we know that Xiaohongshu has recently seen an influx of foreign users, and one of their major needs is to come up with Chinese names. "Can Step Reasoner mini help them?
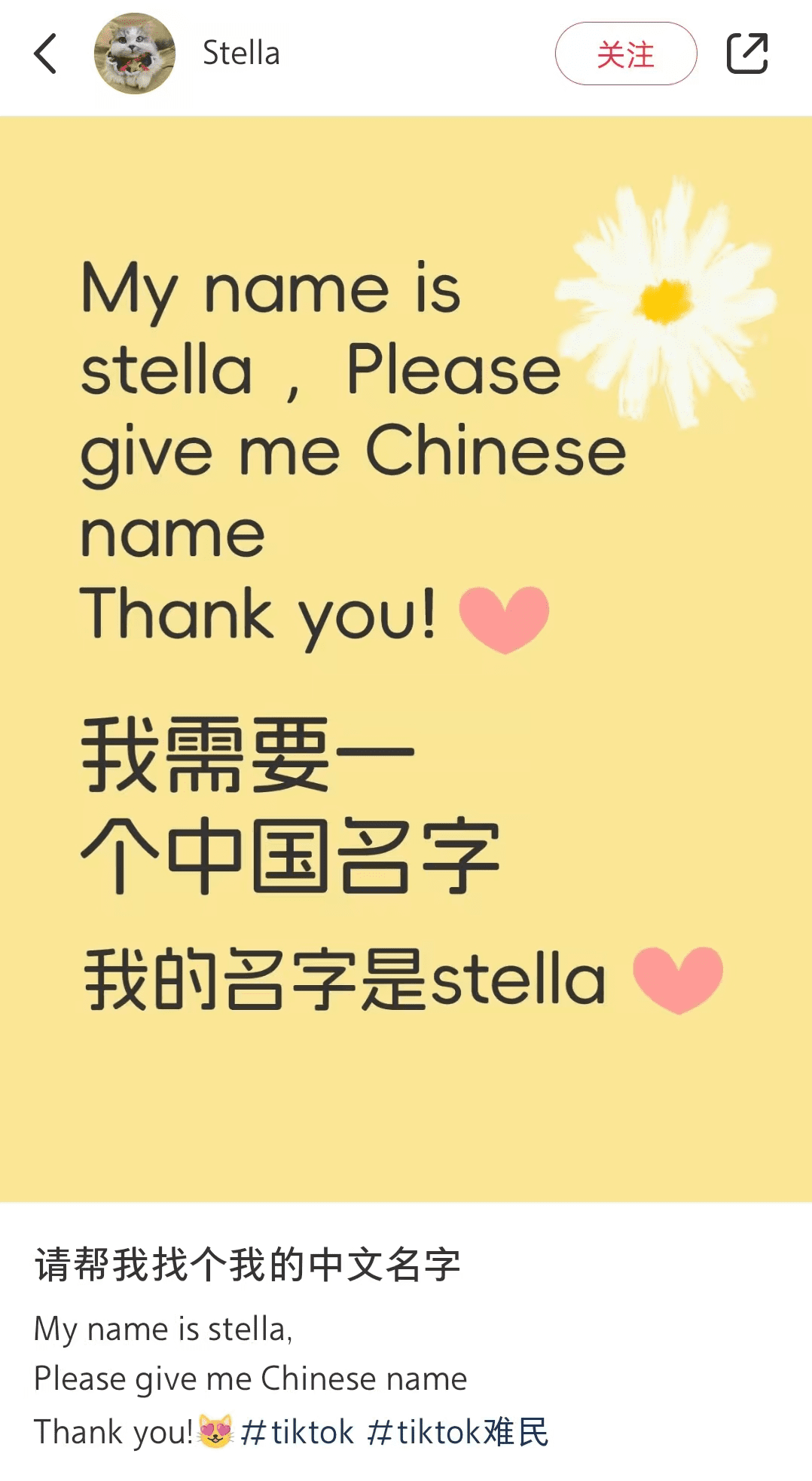
As you can see, for English questions, Step Reasoner mini can smoothly switch to English thinking mode, while still interspersing thoughts on Chinese meanings. As for the name "Starry" that it finally got, it still feels good.
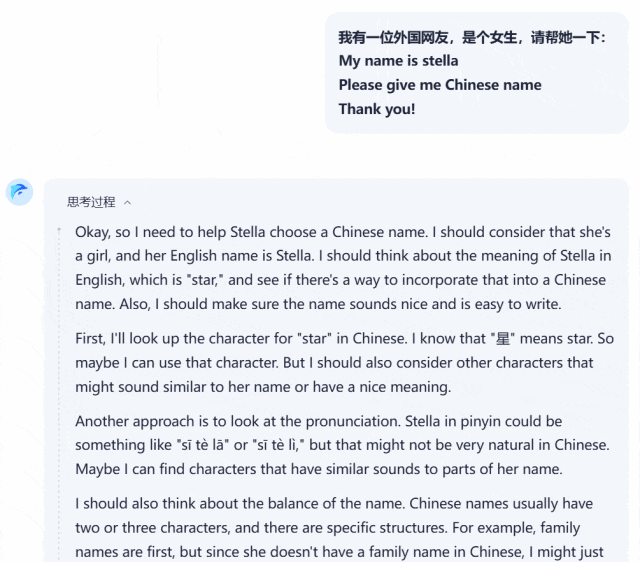
Egg: Visual Reasoning Models are on the way, too!
From today's announcement, in addition to the verbal reasoning model, Step Star also released a small egg: it is building a visual reasoning model to incorporate reasoning capabilities into a larger model with more interactive forms.
For the Reasoning problem in complex visual scenes, Step Star introduces slow perception andspatial reasoningThe idea is to transfer Test-Time Scaling from textual space to visual space and realize Spatial-Slow-Thinking in visual space.
How effective is it? See the display:
1. Answer the questions in the figure
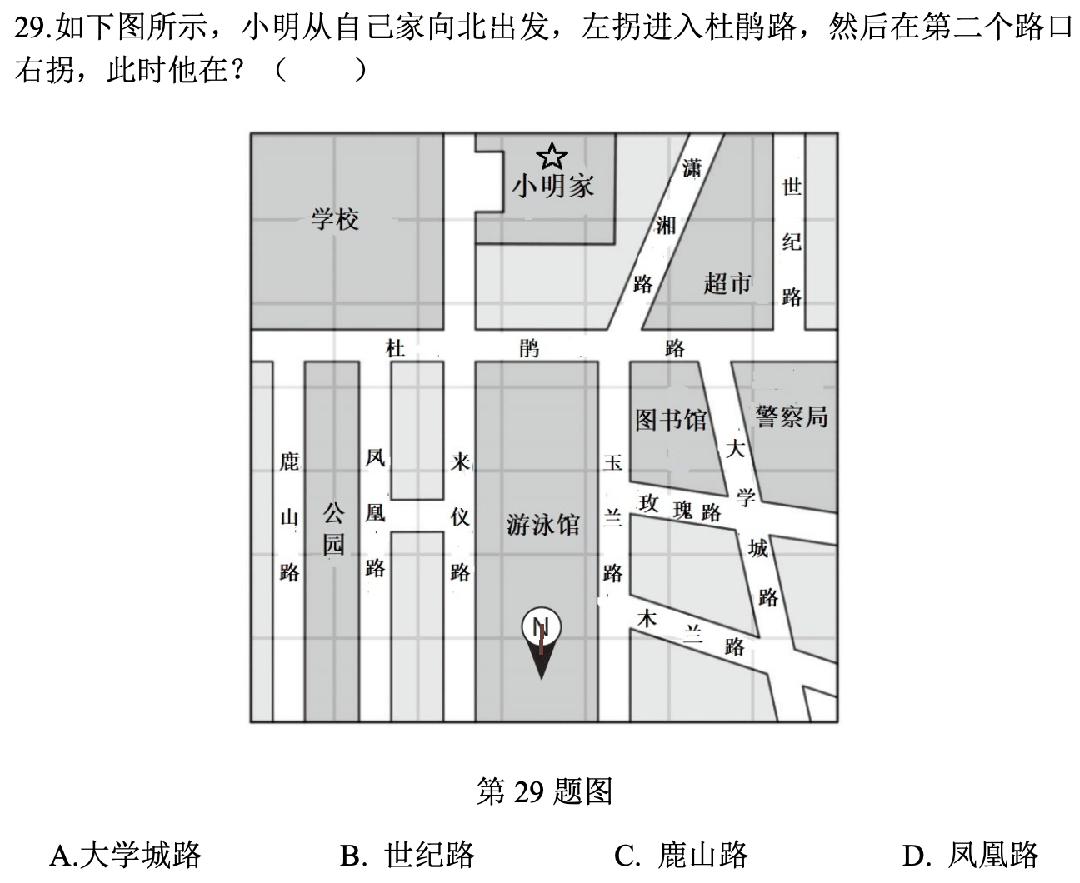
2. Which one can I reach from the blue arrow?
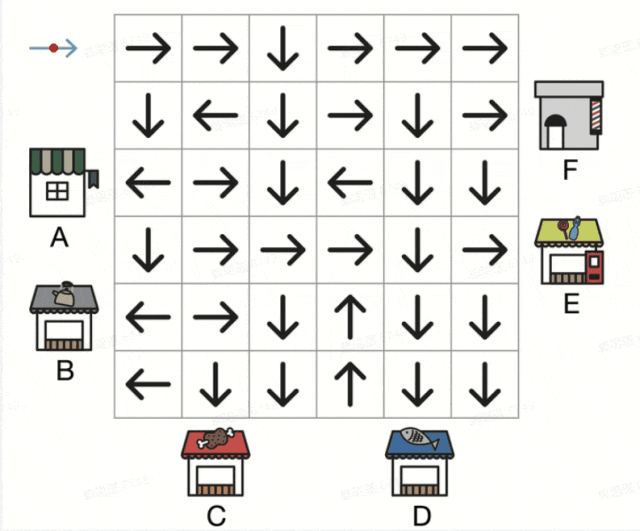
3. What are the numbers corresponding to each of these balls?
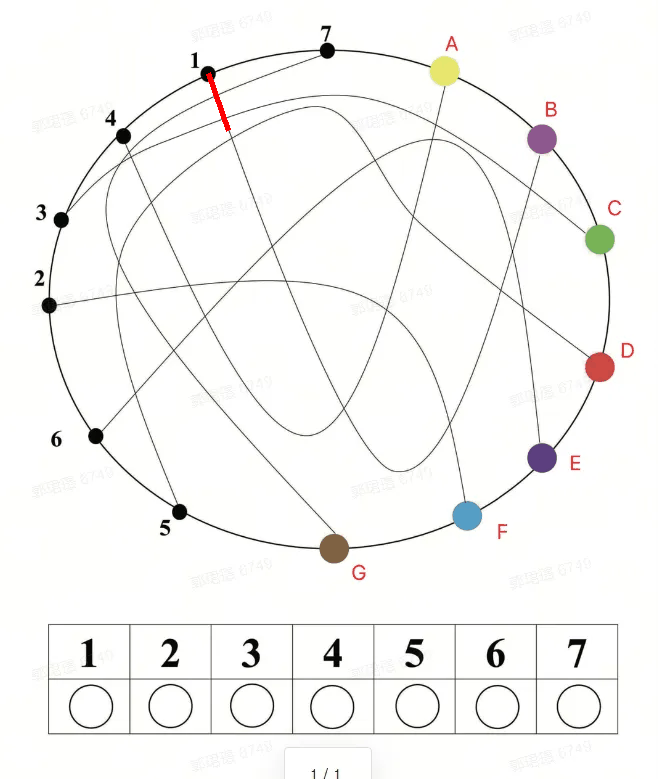
© Copyright notes
The article is copyrighted and should not be reproduced without permission.
Related posts
No comments...