Making predictions, especially in a fast-moving field like data and AI, is notoriously difficult. Nevertheless, we.Rajesh Parikh cap (a poem) Sanjeev Mohan, last year released our Trend forecast for 2024. As 2024 comes to a close, we are pleased to confirm that our predictions were spot on. This success is all the more noteworthy given the unprecedented pace of AI development, a rate of change that is rare in the IT industry.
In our top four predictions, we emphasized the rise of intelligent data platforms and AI intelligences. While these trends are less obvious in 2023, the momentum behind AI intelligences is now undeniable, heralding further acceleration. the mainstreaming of AI and AI intelligences continues.
On the data platform side, we are observing a strong shift toward intelligent, unified platforms, driven by the need to simplify the user experience and accelerate data and AI product development. This trend is expected to intensify as more vendors enter the market and expand the range of options available to organizations.
Expectations for 2025
As we move toward 2025, the landscape of enterprise data and AI will undergo a major transformation, reshaping industries and redefining how humans interact with technology. Rather than calling this a prediction, we want to use this document to explore these transformative trends that we believe require close attention from enterprise executives and technology managers. As such, readers should use it as a guide for setting priorities and preparing their organizations to choose the right direction.
Without further ado, let's dive into the trends we believe are likely to dominate the landscape of enterprise data and AI. Figure 1 illustrates the trends categorized as Applied AI, Data & Ops, and Moon Programs.
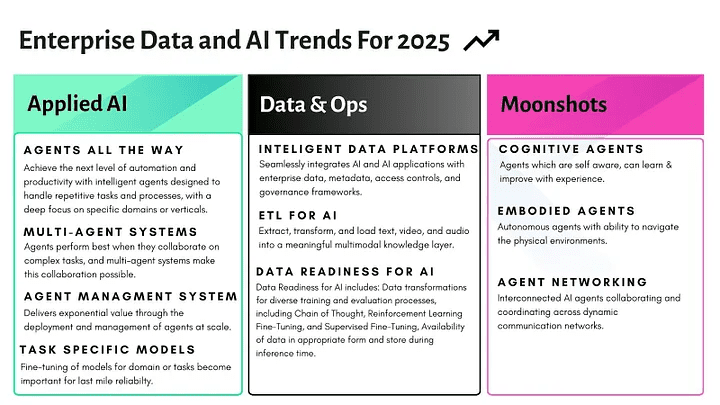
Figure 1: The data and AI landscape of 2025 is characterized by the rise of intelligences, the evolution of data platforms, and the pursuit of ambitious plans to land on the moon that have the potential to transform the world around us.
- Applied AI: These trends will significantly impact how organizations leverage AI models for transformation, especially in terms of how intelligences automate everyday tasks and functions. As models continue to advance in their reasoning capabilities, these intelligences will evolve to handle increasingly complex tasks and collaborate seamlessly.
- Data and platform trends:Converged data and metadata planes that support structured and unstructured data will drive AI and serve as the foundation for intelligentsia and AI applications. A number of key trends are converging to support this vision, including advances in data platform management and the development of robust middleware for intelligent body applications.
- Moon Landing Program:These ambitious, high-risk attempts push the boundaries of current technology and explore areas that may seem cutting-edge today. While there is a high risk of failure, breakthroughs in these areas have the potential to revolutionize the industry and redefine human-computer interaction.
Applied AI
2025Applied AI Trends are centered around practical applications and mainstream adoption of intelligences. As shown in Figure 2, we have identified four key sub-themes that are expected to have the most significant impact in this category.
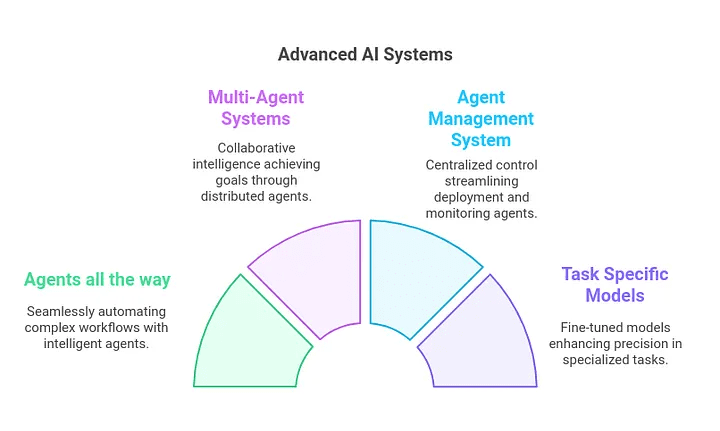
Figure 2: Like machine learning, the democratization of AI will be achieved through a thriving ecosystem of intelligences that can address a variety of tasks and domain-specific challenges.
Next, let's review the trends for each AI/AI application.
Intelligent bodies are everywhere
In 2025, we will enter the era of intelligent body AI.
Here's an excerpt from last year's Trends about AI intelligences and our advice for businesses.
We see AI-informed intelligences as a trend that may take years to realize; however, given its promise, we expect 2024 to be the year of significant progress in intelligences infrastructure/tooling as well as early adoption. It should be noted that much of our understanding of the potential of current AI architectures to take on more complex tasks is still largely about potential, and there are considerable unresolved issues.
Nonetheless, the organization must commit to a pragmatic approach to building intelligent body applications and somehow expect that the gap between current AI technologies in taking on more and more complex automation is likely to close year on year. It must also consider the extent to which automation will be realized on a use case by use case basis over the next 12 months. The evolutionary path/journey of such projects may be more successful in such endeavors.
In 2025, the adoption of intelligent autonomous AI intelligences in the enterprise is expected to accelerate due to the growing need to automate repetitive tasks and enhance customer experience. These intelligences will augment human capabilities and enable us to focus on creative, strategic, and complex work.
They extend automation to tasks that require high levels of thinking, reasoning and problem solving - tasks that currently require significant human involvement. For example, intelligences can conduct market research, analyze data, or answer customer support queries. They can also automate complex, multi-step workflows that were previously considered impractical due to complexity, cost, or both.
An AI Intelligence is a program or system that can perceive its environment, reason, break down a given task into a series of steps, make decisions, and take action to autonomously complete those specific tasks, much like a human worker.
We're currently witnessing the emergence of AI-driven tools, such as developer co-pilots that can be used for about $20 a month, and tools like the Devin Early-stage intelligences such as these priced at $500 (which still represents a Level 2 automation solution.) Level 2 AI intelligences are those that can perform certain tasks autonomously, but still require significant human oversight and intervention.
However, in 2025, we expect to see more advanced intelligences with correspondingly higher prices to reflect the value they provide. For example, a specialized smartbody that can outperform a junior marketer in developing a department's top-of-funnel inbound and outbound marketing strategy could cost as much as $20,000.
multi-intelligence system
Multi-Agent Systems (MAS) enable multiple autonomous intelligences to work together, communicate, and collaborate to solve complex challenges that would be insurmountable for a single intelligence.This specialization within the MAS allows each intelligence to focus on its area of expertise, thereby increasing the overall efficiency of the system as the intelligences contribute their unique skills and knowledge to solve complex problems. These intelligences interact with each other, often using different communication modes and channels, to achieve their individual goals or overall system goals.
Figure 3 illustrates how multiple intelligences can collaborate to enhance content generation within an organization.
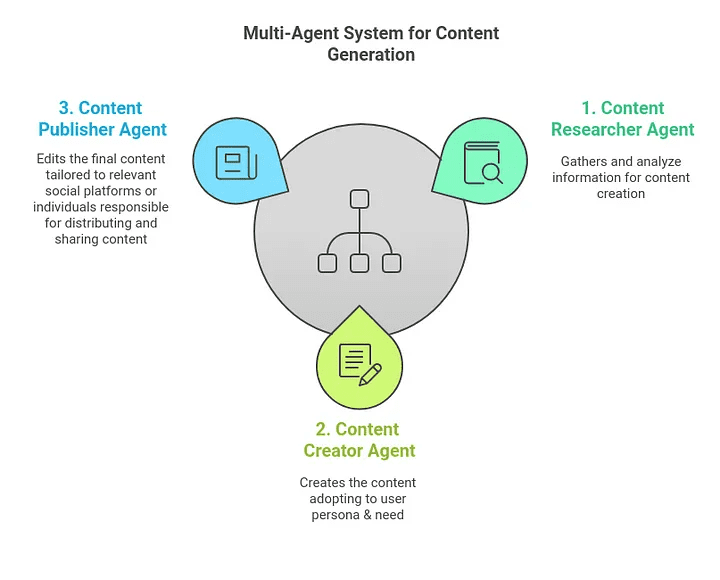
Figure 3: A multi-intelligence system consisting of three intelligences that focus on content research, creation, and distribution tasks, working collaboratively to meet the needs of the marketing department.
MAS can demonstrate different levels of control and different architectural patterns for communication and coordination through common architectural patterns:
- Layered teams:This type of MAS typically uses a central manager or task delegator to mediate communication. Worker intelligences within the system communicate only through this central intelligence, thus preventing direct communication between intelligences.
- Peer-to-peer:In peer-to-peer MAS, intelligences communicate directly with each other without relying on a centralized agency.
- Group Collaboration:This type of MAS is similar to group chats (e.g., Slack, Microsoft Teams) in which intelligences subscribe to relevant channels and are coordinated through a publish-subscribe architecture.
Unlike single-intelligent-body systems, in which a single intelligent body handles multiple roles, MASs allow for efficient specialization, which improves the performance of a wide variety of applications.MASs are critical for scaling complex intelligent-body automation; loading too many tasks into a single intelligent body introduces complexity and scalability/reliability issues.
We foresee a trend for companies to develop more specialized intelligences. These intelligences must operate in team configurations, collaborating and coordinating to enable larger, more complex workflows. As a result, MAS will play a key role in the overall success of intelligence-driven workflow automation programs.
Intelligent Body Management System (IBMS)
An Intelligence Management System (AMS) facilitates the development, evaluation, deployment, and post-deployment monitoring of AI intelligences. By simplifying the creation and refinement of these intelligences, AMS enables faster iteration and simplifies lifecycle management. It also ensures that intelligences meet expectations through comprehensive pre-deployment testing and continuous production monitoring.
Figure 4 shows the components of a representative AMS.
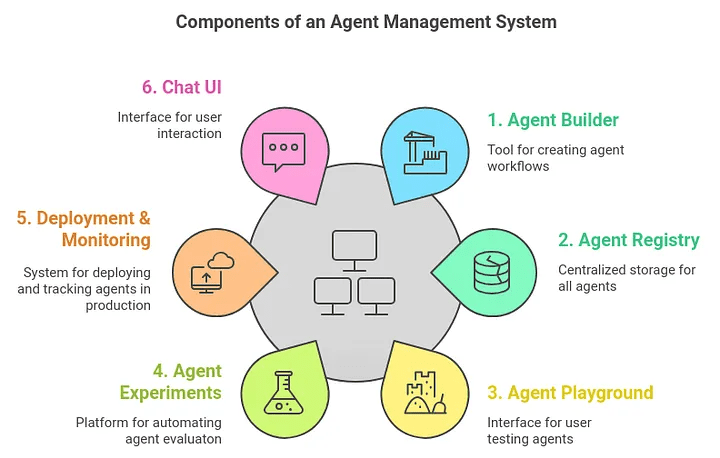
Figure 4: Components of a representative intelligent body management system (AMS)
A representative AMS contains the following components:
- Intelligent body builder:Intelligent body builders, often referred to as intelligent body frameworks, help to quickly create new intelligent bodies and iteratively improve existing ones.
- Intelligent Body Registry:The Smartbody Registry maintains a catalog of available Smartbodies and facilitates access control and governance, which incorporates versioning to ensure appropriate access for the target audience.
- Smart Body Playground:Intelligent Body Playground for manually operated Testing the performance of intelligences in a variety of tasks and user queries provides a user-friendly plug-and-play interface. This environment allows for rapid evaluation of intelligence performance.
- Intelligent body experiments:Smart Body Experimental Support automation Perform a pre-deployment assessment of intelligences. This structured approach evaluates the performance of intelligences by defining the dataset, selecting appropriate metrics, configuring the environment, analyzing the results, and generating an evaluation report. Logs of previously run experiments are often available as well.
- Deployment and monitoring:Intelligent body deployment involves configuring the necessary resources for the intelligent body in a staging or production environment, while monitoring keeps track of relevant runtime metrics. This ensures the reliability and effectiveness of the intelligences.
- Chat UI:The Chat UI provides the necessary user interface required to interact with intelligences deployed in a production environment.
We anticipate that enterprises will deploy a large number of purpose-built intelligences to address a variety of domain-specific tasks.AMS will play a key role in supporting organizations in creating, deploying, and managing these intelligences across the entire lifecycle, leading to an intelligences-enabled enterprise.
task-specific model
(go ahead and do it) without hesitating Anthropic Claude, the OpenAI GPT family, Google's Gemini and leading models such as AWS' Nova dominate in 2024, but there are some noteworthy trends in the development of task- and domain-specific models that are particularly relevant to enterprise use cases.
Figure 5 illustrates the steps involved in this model creation process. This process is commonly referred to as post-training alignmentThe
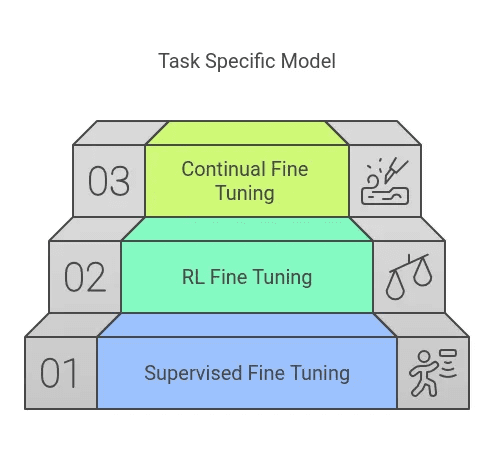
Figure 5: Techniques used to create the domain model
1. Supervisory fine-tuning
Supervised fine-tuning (SFT) involves training a base model (usually a pre-trained base model or a command-tuned variant) using a preference dataset. In the thought chain (CoT) aligned context, each record in this dataset typically contains a triple (Prompt, CoT, Output) where the CoT explicitly references the relevant security specification.
The Contextual Refinement process creates a dataset, starting with a model trained only for usefulness and prompting it with a safety specification and relevant hints. The result of this process is SFT modelThe
2. Enhanced learning fine-tuning
The second phase uses high computational reinforcement learning (RL). This phase uses judgmental LLM to reward signals based on the model's adherence to the safety specification, thus further improving the model's ability to reason safely. Crucially, the entire process requires minimal human intervention beyond initial specification creation and high-level evaluation.
CoT reasoning allows the LLM to explicitly express its reasoning process, making its decisions more transparent and interpretable. In the RL phase alignment, the CoT includes references to the safety specification that describe how the model arrived at its response. This allows the model to carefully consider safety-related issues before generating an answer. Including the CoT in the training data allows the model to learn to use this form of reasoning to obtain a safer response, thus improving safety and interpretability. The output of this phase is often referred to as "Reasoning Models"
3. Continuous fine-tuning
Continuous fine-tuning enables AI engineers and data scientists to adapt models to specific use cases. Deep learning engineers and data scientists can now fine-tune cutting-edge and open-source models using 10 to 1,000 examples, significantly improving model quality for targeted applications. This is critical for organizations looking to improve the reliability of their use-case-specific models without having to invest in extensive post-training infrastructure.
Most cutting-edge models now offer continuous fine-tuning APIs for preference tuning and reinforcement learning fine-tuning (RLFT), lowering the threshold for creating task- or domain-specific models.
The output of this phase can be called "Task- or domain-specific models"The
Open source fine-tuning frameworks (e.g., Hugging Face Transformers Reinforcement Learning (TRL), Unsloth, etc.) provide similar continuous tuning capabilities for OSS models. For example, early adopters of the Llama model have fine-tuned it more than 85,000 times since its release.
As we continue to roll out AI adoption in the enterprise, we observe two distinct trends here:
- Organizations with significant capital resources (which we call "Frontiers") may employ a strategy of post-training open-source models, customizing them extensively for specific domains and use cases through continuous fine-tuning.
- For those with limited budgets but a high focus on reliable use casesAmbitious businessA cost-effective strategy is to choose off-the-shelf LLMs and prioritize task-specific alignment through continuous fine-tuning.
Data and Operations Trends
Data is critical to successful AI implementations and requires data management best practices. Figure 6 illustrates key data and O&M trends for 2025.
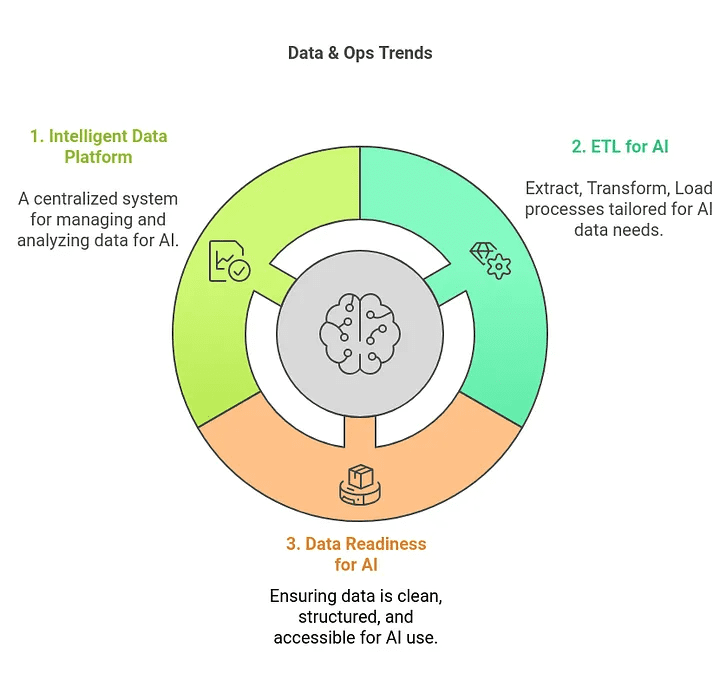
Figure 6: Key Data and O&M Trends
Let's dive into each trend.
Intelligent Data Platform
To accelerate data and AI innovation and reduce operational overhead, we propose a unified Intelligent Data and AI Platform (IDP) in 2024. This unification and simplification effort has gained significant traction among major software providers, resulting in the architecture shown in Figure 7.
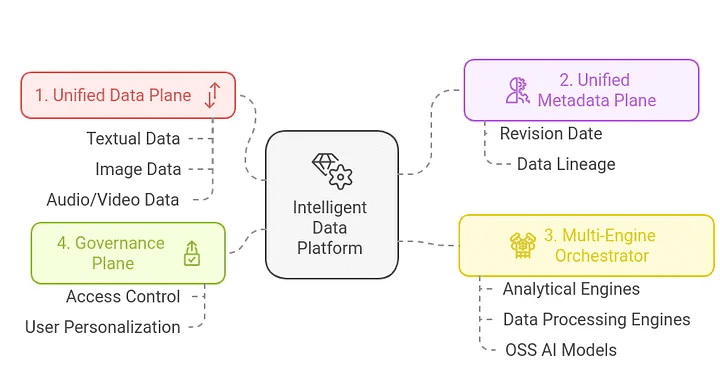
Figure 7: Smart Data Platform Architecture
IDP streamlines the integration of the data lifecycle (storage, processing, analytics, and machine learning), thereby reducing the need for fragmented tools and labor. It also provides a centralized framework for data governance strategy and execution.
While major offerings from both established tech companies and startups continue to enhance functionality in 2024, widespread adoption of data and AI platforms for AI intelligences is still a work in progress.
In 2025, data platform providers will continue to integrate their services to create a critical foundation for AI intelligences and multi-intelligence systems, providing these applications with the information they need to operate and make decisions. These platforms extract three key functions:
- Harmonization of data planes:The Unified Data Plane supports the loading, storage, management, and governance of a variety of data formats, including text (e.g., PDF), images (e.g., PNG, JPEG), and audio/video (e.g., MP3). A key sub-trend in this unified data plane is the adoption of open table formats such as Apache Iceberg, Delta Lake, and Apache Hudi.
- Harmonization of the metadata plane:Metadata provides AI applications with basic contextual information about the data they process. For example, if the data contains an HR policy document, relevant metadata might include the document's version number, last revision date, and author. Without rich metadata that provides these nuances, it will be difficult for intelligences to establish sufficient context and provide the intended functionality.
- Multi-Engine Orchestrator:The IDP also provides a scalable orchestration layer designed to manage and orchestrate various compute engines, including those used for analytical processing, data transformation, and execution of AI models.
- Governance plane: IDP also serves as access control, governance, and personalization middleware, enabling intelligences to better understand user roles (including roles, data access, and query history) and personalize responses.
ETL for AI
ETL (Extract, Transform and Load) is a key data integration process used to prepare raw data for AI and machine learning models. This process involves extracting data from a variety of sources, transforming it through cleansing and formatting, and then loading it into a data management or storage system, such as an IDP, data warehouse, or vector store as described earlier.
While organizations are already familiar with ETL for structured data (extracting, transforming, and loading data from operational databases into warehouses or data lakes), ETL for AI extends this process to encompass a wide variety of data formats, including text (.pdf, .md, .docx), audio/video (mp3, mpeg), and images (jpeg, png).
These unstructured data sources may include a variety of content repositories, applications, and Web resources used by the enterprise. In fact, the ETL process itself can leverage AI for extraction tasks, such as extracting entities (images, tables, and named entities) from PDFs using multimodal Large Language Models (LLMs) or Optical Character Recognition (OCR) models.
ETL for unstructured data Support for a variety of downstream use cases:
- AI-driven insights:Search Enhanced Generation (RAG) enables applications to facilitate user interaction with documents, extract key summaries and support similar use cases. Extracting and transforming data from sources as diverse as SharePoint, Dropbox, Notion and various cloud repositories and applications will be a key enabler of AI-driven insights. We anticipate that vendors will continue to extract RAG and integrate it as a readily accessible feature in unified data, analytics and AI platforms.
- AI Search: Improved accessibility and intelligence of enterprise content compared to traditional keyword searches.
- AI-driven automation: Provide the necessary knowledge layer from unstructured data to provide basic contextual information to intelligences.
- Post-training alignment and continuous fine-tuning: Facilitate the availability of new and updated data to enable seamless and continuous personalization of models for a variety of departmental use cases.
Data preparation for AI
Data preparation is fundamental to the successful implementation of task-specific models and AI intelligences.
This is the key to the success of such programs"precondition".
In order to make data available for AI, it needs to be prepared comprehensively across multiple dimensions. While AI can consume almost all of the available data an organization has, the right approach is to derive data preparation requirements from the use cases being prioritized.
Figure 8 illustrates some of the key dimensions of data preparation for AI
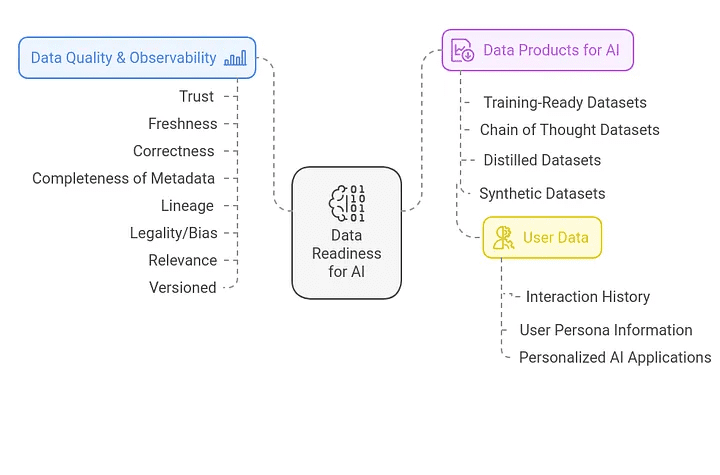
Figure 8: Data Preparation for AI
Data quality and observability
Does this data pass the established quality indicators? This could mean one or more of the following:
- trust
- freshness
- correctness
- Integrity of metadata
- descents
- Legality/deviation
- relevance
- versioning
How are the above metrics managed, tracked and presented in real time?
- Observability data
- Data lineage
- revision history (of a document, web page etc)
Data products for AI
Data products are critical to the success of task-specific models, benchmarking and testing of AI models and intelligent body applications. Some important AI data products include:
1. datasets available for training: Labeled data becomes a valuable data product that can be used immediately for AI training.
2. Chain (CoT) dataset:Unlike traditional datasets, which typically provide inputs and outputs for training, CoT datasets also include intermediate inference steps that explain how answers were derived. This step-by-step reasoning approach is closely related to the way humans solve complex problems, making CoT datasets valuable for training AI models to perform tasks that require logical reasoning, planning, and interpretability.
3. Refined dataset:Provides a smaller representative subset of the dataset that captures the diversity and variability of the full dataset. Below are a few examples of distilled datasets:
a. A subset of customer reviews capturing different sentiment levels and product categories.
b. High-quality task-specific datasets created to train smaller models (student models) to mimic the performance of larger, more complex models (teacher models).
c. A subset of technical documentation refined for fine-tuning the technical question-and-answer model.
4. Synthesized data sets:Refined data used to generate synthetic datasets that mimic the core attributes of the original dataset. They are often used to augment real datasets where data is scarce or unbalanced. By generating variants, models can be trained on more diverse datasets.
5. Knowledge mapping datasets:Data products powered by GraphRAG utilize graph-based data retrieval and generation capabilities. For example, healthcare knowledge graph datasets connecting medical terminology, diagnoses, treatments and patient outcomes can be used to provide personalized medical advice, suggest possible treatment options and help physicians make data-driven decisions.
6. User data:User data is critical in building smarter, personalized AI applications. This data typically includes any information about the user's role and the user interactions or inputs that the AI intelligence or application uses to understand the user's role (which the intelligence can utilize to provide meaningful outputs or responses). Here are some examples of user data:
a. Data analyst intelligences with user roles and information about user dataset/query/dashboard interaction history can personalize query responses by filtering and selecting appropriate dataset access histories and query runs.
b. Customer support intelligences that understand a user's customer status (e.g., Premium or Normal) and the nature of past support requests can use past work orders, problems, and solutions to prioritize responses, provide faster solutions, or recommend specific knowledge base articles.
c. By analyzing past communication history and engagement patterns with prospects and customers, sales intelligences can personalize follow-up strategies, recommend specific products or services, and prioritize prospects based on their historical behavior.
moon landing program
Moon landing programs are ambitious, exploratory attempts to solve major challenges through breakthrough solutions. These programs typically push the boundaries of current technology and operate at the cutting edge of innovation. While they inherently carry a high risk of failure, the potential for transformative outcomes is enormous.
While this section is a space for creative exploration, we would like to explore the more speculative concepts highlighted in Figure 9.
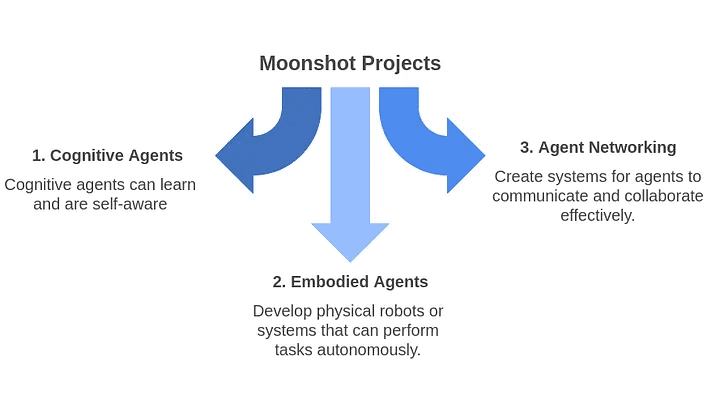
Figure 9: Moon Landing Program
cognitive agent
Cognitive intelligences learn from their experiences extremely and continuously, and adapt and improve. Figure 10 depicts the defining characteristics of the cognitive intelligences.
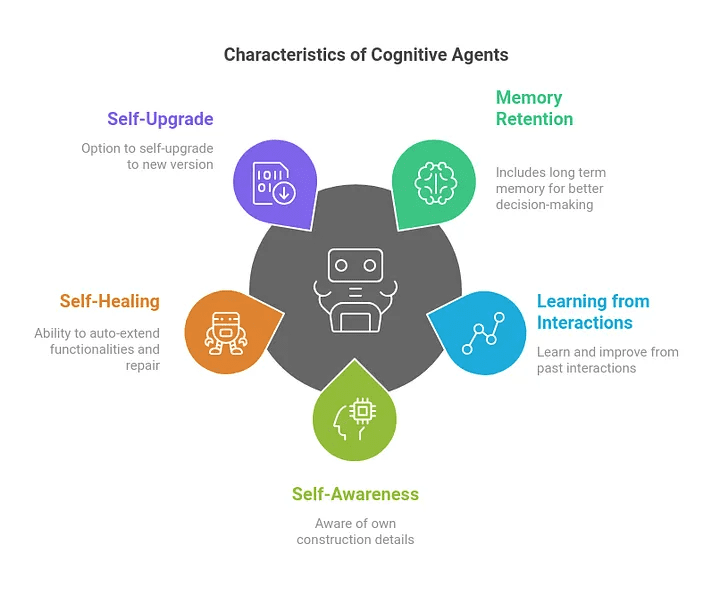
Figure 10: Five key features of cognitive intelligences: learning, memory, self-awareness, self-improvement, and self-escalation
In addition to the generalized capabilities of AI intelligences, cognitive intelligences typically have several other capabilities:
1. Memory retention
Longer memory retention capacity is one of the key characteristics of cognitive intelligences. Memory retention capacity allows intelligences to recall previous conversations, often remembering specific events, including when and where they occurred, and often learning from them.
Thus, cognitive intelligences have complex memory architectures that include long-term storage for retention and specific forms of memory (e.g., situational memory), which allow intelligences to recall and recall specific events in time, including when and where they occurred.
An example use of situational memory could be to recall the steps taken to successfully complete a task in a previous event. If the intelligent body is faced with the same task again, it can recall the exact steps taken in the previous successful instance and perform that task more efficiently this time.
2. Learning from past interactions
These intelligences learn from past interactions and use that learning to make better decisions in the future.
3. Self-awareness
These intelligences may also be aware of their own construction details and functions.
They can potentially learn from user interactions and update their knowledge base for new learning content.
4. Self-healing
Self-healing enables the Intelligence to extend its functionality by adding new features, such as tools to create preference datasets from recent interactions and trigger the next fine-tuning job. It can also further evaluate new models and register new model revisions in the model registry while generating detailed model reports for AI engineers to review.
5. Self-escalation
(Optional) Intelligentsia may self-upgrade to the new model revisions created above.
embodied intelligence
Embodied intelligences are types of AI intelligences that have a physical presence (e.g., robots). This "embodiment" is critical because it allows the intelligence to perceive and act on the physical world as a human would, enabling it to learn and perform tasks that require the intelligence to develop a deep understanding of the physical space and perform assigned tasks. Generative AI is expected to revolutionize robotics by going beyond traditional rule-based programming to operate in more complex and dynamic environments.
Figure 11 depicts how organizations can use these new intelligences for a variety of applications.
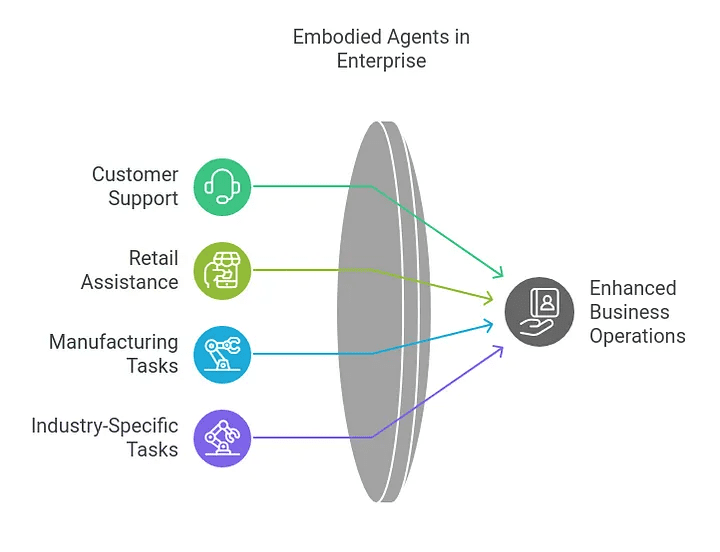
Figure 11: Example of an enterprise using embedded intelligences.
Let's explore how banks are using embodied intelligences. Embodied customer support intelligences in their branches can initiate the first interaction with a walk-in customer, provide personalized financial advice and help process transactions.
In retail, embodied intelligences can take the form of in-store shopping assistants that provide product information and guidance around the store. In manufacturing, these intelligences can handle tasks requiring mobility and dexterity that are dangerous for human safety.
intelligent networking
Effective communication between multiple AI intelligences to achieve common goals or solve complex problems is currently hampered by the lack of standardized message formats, protocols, and conflict resolution mechanisms. Future networking approaches must be scalable, low-latency, and secure, building trust between intelligences and protecting communication networks from malicious attacks.
This brings us to the last expected trend regarding the improvement of smart body networks.
Effective networks of intelligentsia can revolutionize the way intelligentsia communicate, collaborate, coordinate, get work done, and learn, both inside and outside the enterprise. This trend is similar to the early days of the Internet and the standardization of Internet protocols, as well as the evolution of communities and forums in the web 2.0 era. These trends have significantly enhanced collaboration between people beyond physical boundaries.
Figure 12 shows four options for building an effective network of intelligentsia.
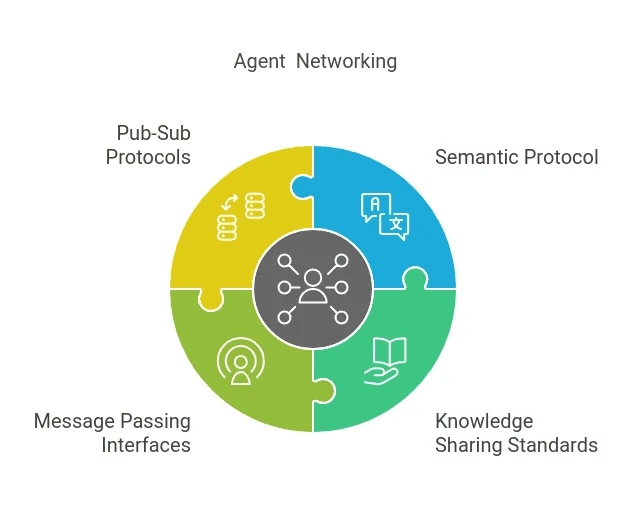
Figure 12: Examples of possible smart body network standards
The benefits of all the moon landing program trends are enormous. Cognitive intelligences can learn through these interactions by analyzing exchanged data, updating their own knowledge base, analyzing exchanged data, improving human-like communication, and accelerating innovation within the boundaries of the enterprise.
reach a verdict
In summary, the Applied AI trend is one that accelerates the meaningful adoption of AI intelligences and applications in the enterprise, while the Data and Ops trends provide a solid foundation for supporting and accelerating these intelligences applications. In addition, the Moon Landing program covers topics that may seem radical today, but could have the next transformative impact.
As usual, the purpose of this study is to focus on technological solutions rather than organizational impacts. Autonomous intelligences naturally raise concerns about job losses as AI intelligences are expected to take over repetitive tasks. Organizations need to rediscover the synergy/coordination between people and AI intelligences by critically redefining job roles and creating new ones around creating, managing, and collaborating with AI. This transformation therefore creates another important task as most organizations need to critically enhance and retrain their workforce alongside the AI transformation.
Finally, any breakthroughs in the LLM modeling architecture, solutions that can introduce adaptive knowledge injection in a cost-effective manner, or significant improvements in comprehension and reasoning capabilities have the potential to further impact the potential reality of AI applications.